Stephanie Weiss, University of Siegen
Abstract
Owing to advancing digitalization, consumers have become more fastidious about the story ventures create about themselves. The increased demand regarding the aesthetical representation of storytelling has prompted many entrepreneurs to trust upon more sophisticated forms, such as images and videos, to create an identity with which they aim to appeal to consumers. For research in the field of storytelling, this step of modernization entails innovating the methodological approaches adopted to analyze visual storytelling. Only then, scholars are able to make reliable statements about the influence of visual storytelling on, e.g., sales tracking. The sales giant Amazon has taken a step in this direction by offering its platform-owned ML-web services. In the scope of this article, it is looked at the potential two of these provided services, Amazon Transcribe (Automatic speech recognition) and Amazon Rekognition (Image and video analysis), hold for advancing the research field of storytelling.
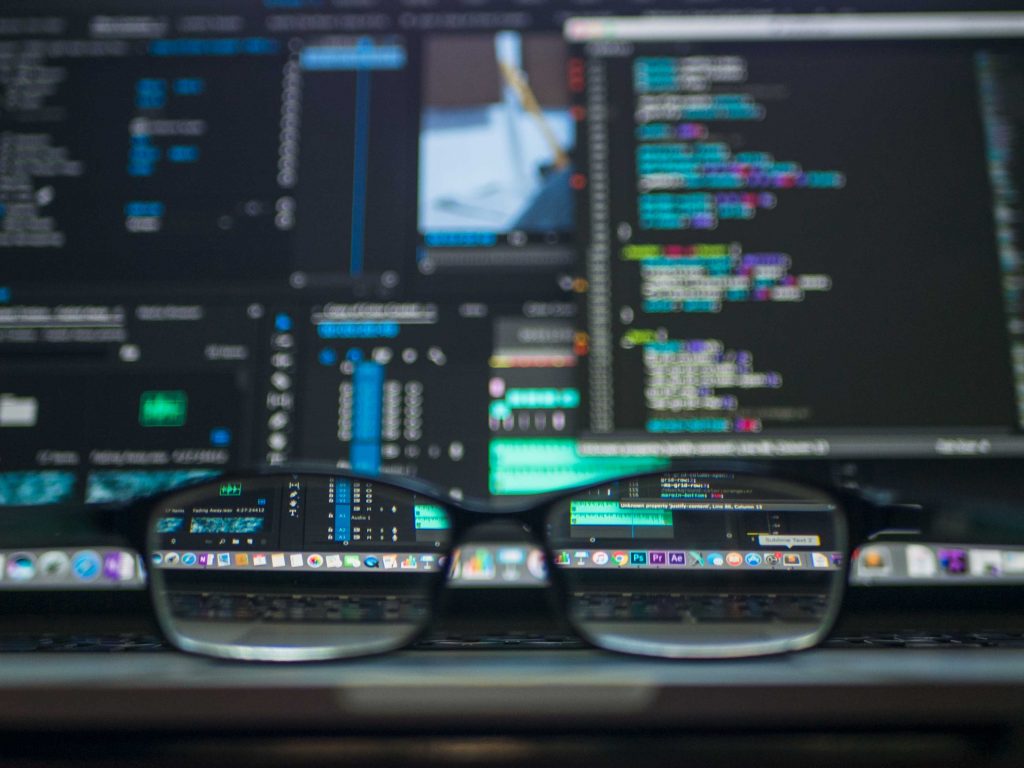
Storytelling – A Powerful, Well-Established Tool
Push messages with image ads pop up on our smartphone screens, video ads interrupt the series we are watching, Facebook & Co. advertise goods we have searched for lately, surrounded by posts of our friends – for those occurrences not to drown in the daily advertisement noise we are experiencing but to make us either focus, sympathize, or laugh, it is important for marketers to master the art of storytelling. Recently, I have watched a very inspiring TEDx Talk by David J. P. Phillips, a man who is known as “Mr. Death by Power Point”, about how this can look like and what is the magic behind storytelling (https://www.youtube.com/watch?v=Nj-hdQMa3uA&feature=youtu.be). Aside from him, there are numerous other supporters who attach a powerful role to storytelling (Figure 2).
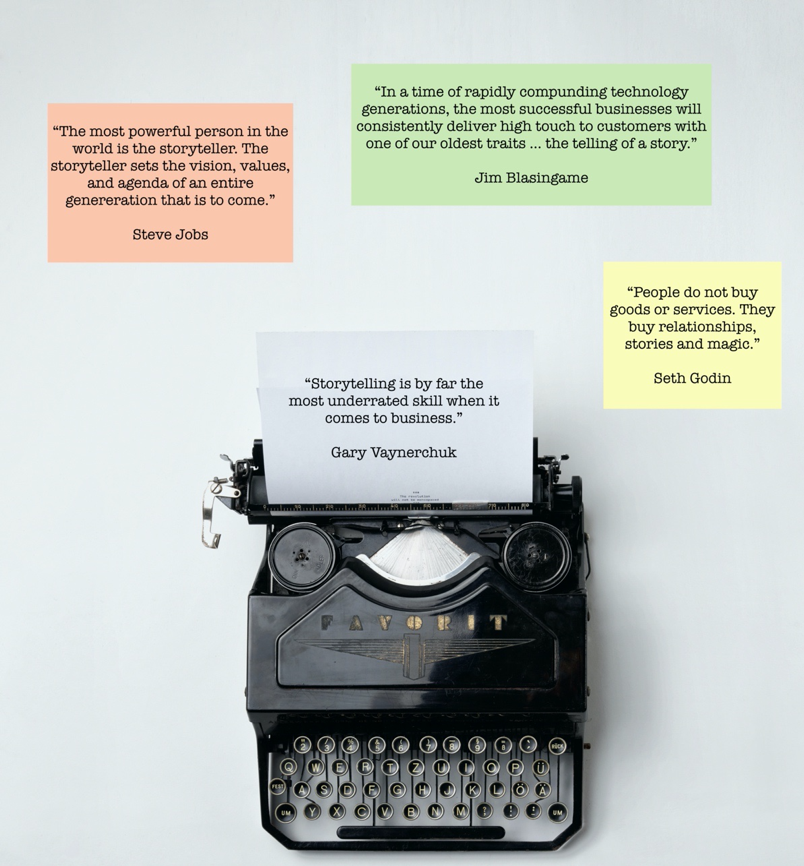
Why storytelling should not be underrated and how ventures can benefit from an effective storytelling has been studied by several scholars (e.g., Lounsbury & Glynn, 2001; Martens, Jennings, & Devereaux Jennings, 2007; Navis & Glynn, 2010). In this article, however, I argue that according to the fact that stories are increasing in complexity regarding the interplay of audio track, images, music, etc. (Pohlgeers, 2019), it is time for a methodological revolution in the field of narratives, e.g., to get a better understanding of the impact they have on sales success. By doing so, I aim to push forward and contribute to visual storytelling as under-researched field. For this purpose, I want to introduce innovative methods and discuss their potential as well as their weaknesses for the analysis of storytelling, contributing to modern entrepreneurship research.
Not only does storytelling work in an entrepreneurial context, but also scholars, to whom I count myself, have to tell a compelling story with their research papers in order to be published. I have been told once by a co-writer that there are three characteristics which make a paper “sexy” and increase its publication chances: (1) new conceptional ideas, (2) an interesting data set, or (3) an innovative method. On the hunt for meeting one of these criteria with one of my research projects, I searched for new avenues in the field of storytelling. During this search, I stumbled upon Amazon Web Service (AWS), a massive platform that provides powerful tools, amongst others, based on artificial intelligence and machine learning, opening up new possibilities and solutions in all kinds of fields, including academic research. Especially the two tools Amazon Rekognition and Amazon Transcribe caught my interest by evoking enthusiasm for new methodological routes I could take in my research field of storytelling. Amazon Rekognition, forming part of facial recognition technologies (FRT), e.g., detects faces, objects, labels, text, safe content, and celebrities in images or videos, and Amazon Transcribe, which converts audio into text, could both revolutionize storytelling research in general and in an entrepreneurial context in particular. By understanding how elements involved in storytelling such as narratives, visuals, and images serve as mechanism to form a venture’s identity (Gehman & Soublière, 2017), researchers could, on the one hand, make valuable contributions to legitimacy literature; on the other hand, they could advise ventures how to create a compelling identity through storytelling in order to be perceived as legitimate by consumers (Martens et al., 2007).
Why Are New Methods Needed in The Field of Entrepreneurial Narratives?
To better account for the power storytelling holds and finding adequate ways to methodologically, grasp the complexity of this today highly mediatized tool, I claim that new methods are needed in the field of storytelling or rather the maturing process of recently emerging methods should be advanced. An emerging methodological revolution already becomes apparent when exploring existing literature in Table 1. In particular during the last two decades, I observe a development from conceptual approaches to natural language processing (NLP). While several studies have examined narrational storytelling, the field of visual storytelling is just starting to become more prominent.
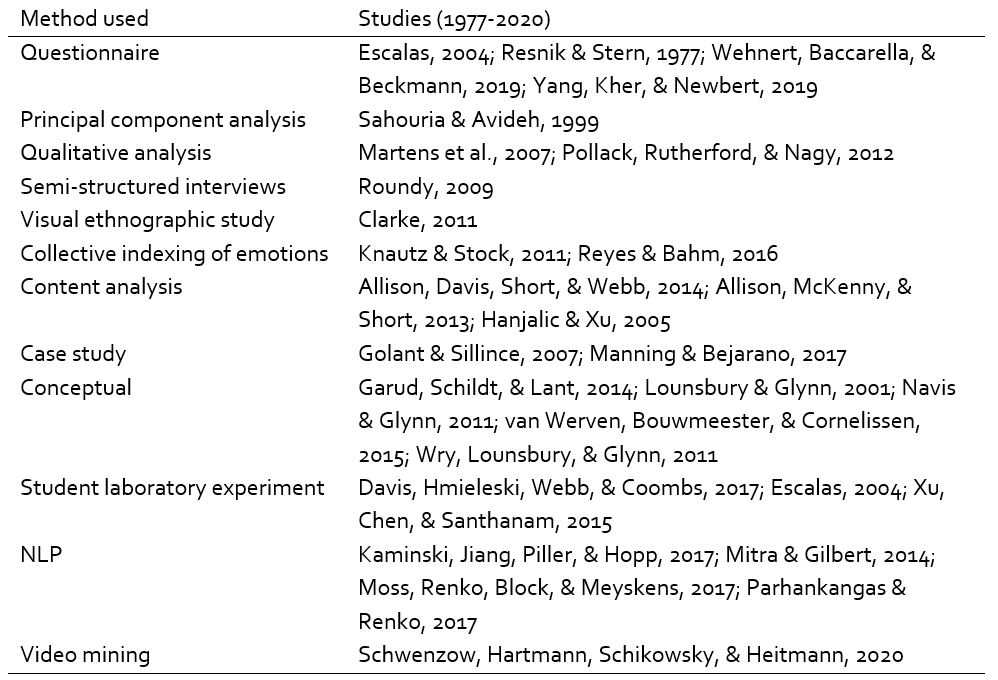
The reasons for new methods which advance research on visual storytelling to create untapped opportunities are twofold. First, innovative methods in this field provide new insights in the phenomenon of storytelling. Second, new combinations of different approaches arise with the aim of gaining a deeper understanding of storytelling and bring together a yet scattered research field (Lounsbury, Cornelissen, Granqvist, & Grodal, 2018). One such combination of approaches could be to investigate the relationship between aesthetics and innovation to gain new insights into sociocultural trends (Jancsary, Meyer, Höllerer, & Boxenbaum, 2018). By aesthetics, existing literature refers to a technological product’s design (Lounsbury et al., 2018). I argue, however, that this interpretation should be extended to a narrative’s design. Another avenue a study about modern entrepreneurship points at relates to research on storytelling as strategic means (Überbacher, 2014) and how multiple linguistic constructs interplay (Lounsbury et al., 2018). To understand the dynamics stemming from this interplay, they motivate research on exploring interactions between contextual factors, such as spaces, places, objects, and language, all factors which scholars could examine with Amazon Rekognition and Amazon Transcribe.
Moreover, I argue that what has already been established in video games user research (Mirza-Babaei, Long, Foley, & McAllister, 2011) could also be promising to overcome the lack of metrics and evaluation methods to measure story effectiveness of new ventures, such as interest stimulation, memorability, and informativeness (Kosara & MacKinlay, 2013) as well as capturing the interplay happening in storytelling. How methods such as video mining as current state of the art provides ways to advance business research in this field demonstrates a recent study in which scholars investigate the appeal of movie trailers (Schwenzow et al., 2020). Relying on biometric data generated through automated processes might, on the one hand, improve replicability of studies when compared to human rating (Chouinard, Scott, & Cusack, 2019), on the other hand, research using machine learning-based algorithms might not be prone to subjectivity issues but instead to error rates. Thus, I assume, at least for an early phase of artificial intelligence (AI), that it might be promising to be a two-timer, combining AI with human crosscheck through, e.g. the use of Amazon’s Mechanical Turk (MTurk) (Chan, Parhankangas, Sahaym, & Oo, 2019). This would allow for visualization studies which are not solely student-based (Kosara & MacKinlay, 2013), increasing their chances of being accepted at journals such as Entrepreneurship Theory and Practice, which abstains from papers with student-based data samples unless the research question shows a clear relevance for consulting students.
Amazon Web Services (AWS) – a Golden Fleece or a Pandora’s Box?
A solely Amazon-based approach, on the other hand, might be a source of new, yet unforeseeable consequences. Hence, a question I am asking myself is whether AWS incarnates a Golden Fleece or a Pandora’s box with the big stirs Amazon as provider of the service platform is creating. These two concepts trace back to Greek mythology where The Golden Fleece is used as a symbol for something valuable with “magic power” and “an object of worship” (Lordkipanidze, 2001, p. 3) that needs to be protected for “whoever owned the Fleece could reign!” (Lordkipanidze, 2001, p. 4). As opposed to this, a Pandora’s box is a proverb we use to refer to “a source of unexpected troubles and pain” (Lordkipanidze, 2001, p. 33). From my point of view, AWS seems to perform a tightrope act with two forces pulling: tools leading to innovative solutions and improvements in diverse fields and a growing monopoly which is involved in a powerful surveillance system, and thus criticized for violating privacy rights, as well as for having momentous error rates and a biased algorithm (Blank, 2019). Before considering the tools provided by AWS for research purposes, I wanted to dig deeper and get an idea of how to estimate these three main concerns coming with the use of FRT in general and through an academic lens in particular.
Facial Data – Privacy Rights as Pitfall
FRT have already entered several areas of our everyday lives. When we transition from one country to another, a snapshot is taken at the airport. Booking an accommodation via Airbnb requires storing our ID card. With our Face ID, we can unlock our iPhone. Facebook uses its “photo tag suggest” tool to identify our friends. Nowadays, this enumeration of FRT application fields seems to be endless. Although these technologies have positive contributions, facilitating our everyday lives, with the advance of FRT also come concerns whether our privacy rights remain unviolated.
As an article by Whitener and Aragon (2019) shows privacy rights’ violation is an explosive issue, reflecting in the General Data Protection Regulation (GDPR) (EU) and the Biometric Information Privacy Act (BIPA) (US). Both regulations distinguish between facial geometry and simple photographs. While the GDPR demands an active consent from the individual whose biometric data should be processed for identification purposes, the BIPA is limited to a mere disclosure duty (Whitener and Aragon, 2019).
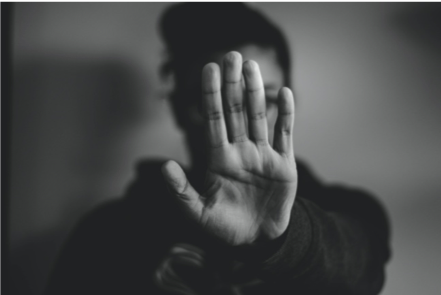
As a study has shown, people are willing to trade benefits for biometric privacy since they are not solely concerned by data security but also by its use (Kugler, 2019). Society strongly criticizes the use of FRT especially in the realm of law enforcement, such as border control and mass surveillance. “Border management is becoming increasingly datalogical” (Mattern, 2018), leading to dehumanizing. “The border-crosser becomes a pixelated subject[s] … composed of so many data points that the individual is no longer a meaningful category.” (Longo, 2017, p. 224). Also, political activists and protesters see this technology as danger (O’Neill, 2019).
The contested deployment of FRT and its potential for mistaken arrests (Fingas, 2018) induced over 100 Amazonians to issue a letter to Jeff Bezos, CEO of Amazon, asking to stop selling facial-recognition software to police and to start taking responsibility (Conger, 2018). The trigger for the request was the disclosure of the collaboration between Amazon, the government, and the police force by the non-profit organization The American Civil Liberties Union (ACLU), which is committed to protecting personal rights. The Amazonians saw a serious threat for marginalized groups through the use of Amazon Rekognition: “We already know that in the midst of historic militarization of police, renewed targeting of Black activists, and the growth of a federal deportation force currently engaged in human rights abuses — this will be another powerful tool for the surveillance state, and ultimately serve to harm the most marginalized” (Conger, 2018).
Male Whites as Standard – An Ongoing Legacy of Slavery and Colonial Times?
Not only is FRT, such as Amazon Rekognition, seen critically due to
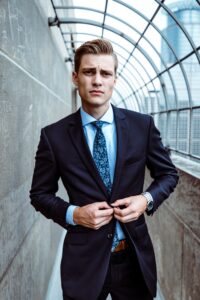
violation of privacy rights, but also it raises concerns with regard to bias (Klare, Burge, Klontz, Bruegge, & Jain, 2012). This brings up the question of the type of bias and who is responsible for it. “Algorithms themselves have no moral agency” (Blank, 2019, p. 6). As a consequence, they are only as good as the training data which their programmers feed them under the influence of their own cultural values. Does that imply that these algorithms do not provide improvements in terms of objectivity? Do they have to be deculturized first?
Since most algorithms, such as, e.g., Microsoft, IBM, Google, and Amazon, have their origin in diversity lacking, American companies (Levin, 2019), Western culture is dominant in AI, which, as results of these algorithms show, marginalizes certain groups, i.e., women, as well as darker-skinned humans, reminding us of slavery and colonial times (Laura & Thomi, 2019; Magnet, 2011). As a study has shown, accuracy of APIs’ results decreases by the following order of training data origin: Caucasian, Indian, Asian and African (Wang, Deng, Hu, Tao, & Huang, 2018). Low cultural distance between developers and the person to be identified improves outcomes of APIs (Beveridge, Givens, Phillips, Draper, & Lui, 2008), supporting the hypothesis that the culture of an algorithm’s origin is dominating. Compared to lighter-skinned males, for darker-skinned females error rates for gender misclassification are up to 34.4% (Buolamwini & Gebru, 2018). This high error rate even led to a classification of African American faces as gorillas by Google’s algorithm (Simonite, 2018).
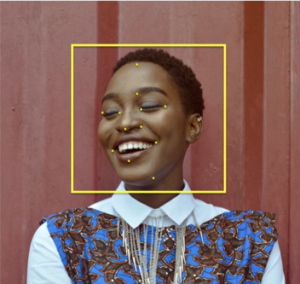
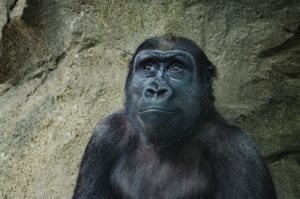
As a study has shown, FRT are not only racist and sexist (Buolamwini & Gebru, 2018) but also age-discriminatory, indicated by an underrepresentation of underaged and elderly (Anda, Lillis, Le-Khac, & Scanlon, 2018). Hence, “biometric technologies not only fail to live up to promises of objectivity and reliability, but in fact continue a long history of misrecognizing women, people of color, and people with disabilities” (Mattern, 2018).
Are Amazon Transcribe and Amazon Rekognition Useful and Reliable?
Apart from concerns regarding privacy rights and bias, a key question that will determine the future of services such as Amazon Rekognition, especially when used for academic purposes, relates to their reliability. To test the usefulness and reliability of Amazon Transcribe and Amazon Rekognition and to exemplify how these tools could revolutionize current methodological approaches in storytelling, I, owing to an own interest in sustainable entrepreneurship, analyzed the following front video of a Kickstarter campaign (alternatively you can go to https://www.youtube.com/watch?v=CxBoTMWQTd0 where only the Kickstarter dedication is missing). The video starts with a waterfall scenery representing the massive amount of water we use for our daily showers. Throughout the video four different subjects (1 man, 2 women, 1 girl) test the innovative, water-saving shower the company produces. The viewer learns about the journey of the company starting from the idea, going through a developmental design and engineering process, resulting in the final product of which water efficiency is demonstrated by the filling levels of two aquariums representing the water consumption during a “normal” shower compared to when using the innovative product. The story about the product’s journey is accompanied by meeting scenes in office rooms covered with sketches and close-ups of the product. Crowdfunding is an interesting environment to investigate visual storytelling since campaigns with a video are much more likely to reach their funding goals, whereas those without a video have a hard time receiving sufficient funding (Kickstarter, 2020).
Amazon Transcribe – from Speech to Text
For a reliability check-up of Amazon Transcribe, first, I closely scrutinized the rendered transcript by comparing it to the original audio track in the sample video. Figure 7 displays the transcript with incorrectly transcribed parts which I emphasized in different colors and added corrections. With the help of these emphases, I evaluate total accuracy, group error types, and discuss consequences for academic analyses. In total, Amazon Transcribe shows an accuracy of 94,92% (incorrectly transcribes 33 out of 649 words (excluding sentence transition issues). Only 73 words have a confidence level below 85%. 26 of these 73 words (35,62%) are actually incorrectly transcribed. The different colors in Figure 7 represent different badness scales. While red-marked parts severely impact the transcript’s meaning (16), yellow-marked parts emphasize minor modifications (14), concerning mainly sentence-transition. I used brownish color to emphasize sample-specific errors, relating to the product’s name (10). I am pleasantly surprised how accurate Amazon Transcribe worked. When assigning the right weight to the confidence values, using Amazon Transcribe might facilitate investigating narratives embedded in videos in the future. Only analyses based on sentence length might be problematic due to the frequent errors occurring with sentence transition. Moreover, accuracy should be tested for different accents since Amazon Transcribe might have problems transcribing non-standard English.
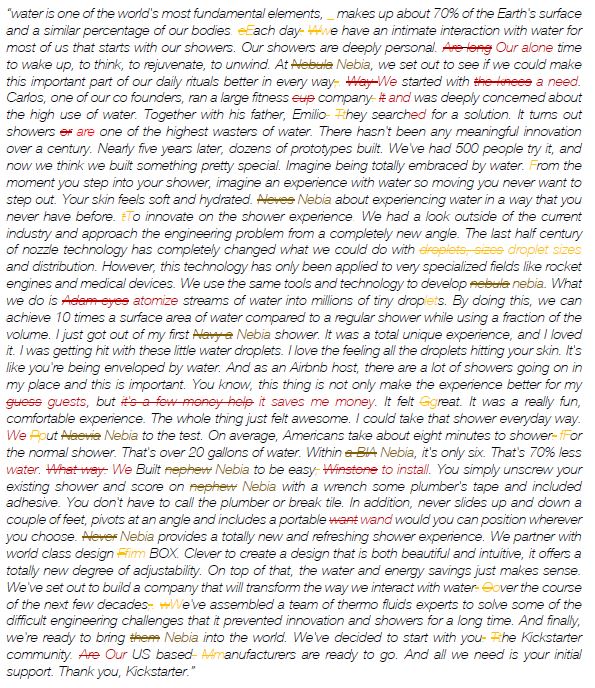
After exploring Amazon Transcribe and its reliability, I turn to Amazon Rekognition. In the scope of this article, I focus on detecting faces and detecting labels as two provided commands. Due to copyright reasons, I took two random pictures (Figure 8 and Figure 9) that could fit the sample crowdfunding campaign I am using to demonstrate what can be done with the tools of interest provided by AWS. For detecting faces, a bounding box with landmarks is created. Based on this face measurement, age, gender, emotions, and other characteristics can be determined. Further information is given about the quality regarding brightness and sharpness as well as the face rotation.
Table 2: Detect faces output for Figure 7
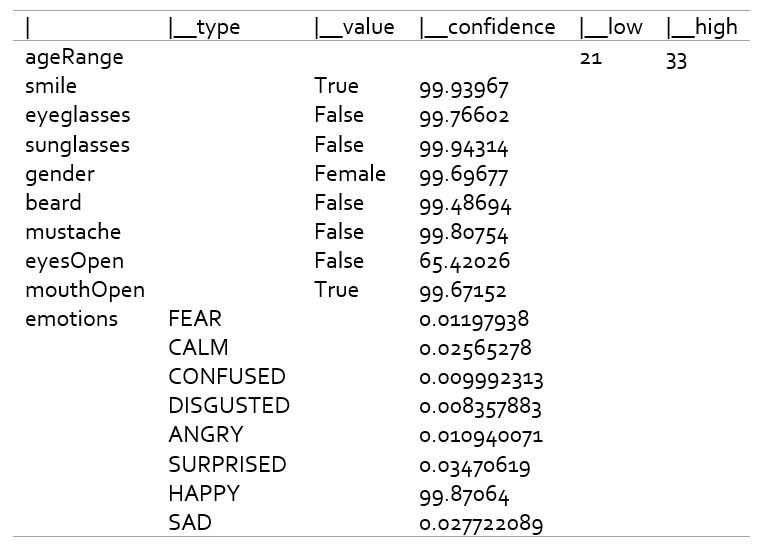
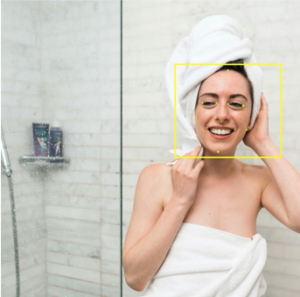
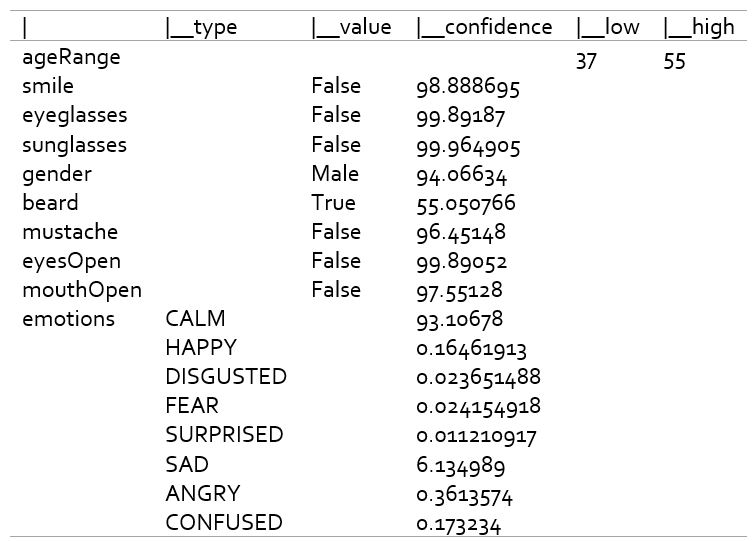
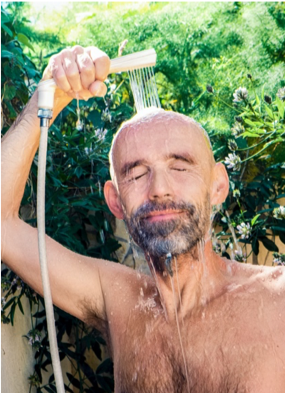
For Figure 8 and Figure 9, results are for the most part reliable. Concerning age, I notice that with increasing age, the age range Amazon Rekognition gives seems to widen. This is in line with prior findings that Amazon Rekognition performs best “for all ranges up to the age of 29” (Anda et al., 2018, p.136). The mean absolute age error estimation is 9.286. To exemplify this, for a woman at the age of 54, the system might render a result of 45. Error rates for males are lower (Anda et al., 2018). Amazon Rekognition’s perfomance for estimating humans at younger ages may already open up new ways to investigate the impact of infant behavior in visual storytelling (Chouinard et al., 2019). Also, results for gender correspond to the accuracy rates identified in recent studies (Jung, An, Kwak, Salminen, & Jansen, 2018; Yavuz, 2019). For emotions, Amazon Rekognition correctly considered happy and calm to be the dominant emotions in Figure 7 and 8 respectively. Being able to identify emotions in pictures will open up new possibilities to advance the field of collective indexing of emotions (Knautz and Stock, 2011; Reyes and Bahm, 2016). Only the confidence level for beard in Figure 8 is surprisingly low.
Amazon Rekognition – Detecting Labels
For the sample video, Amazon Rekognition identifies 4640 labels. These labels refer to detected objects, scenes, and activities. Using the statistical software R, I requested the number of unique labels, which rendered a list of 288 unique labels, as displayed in Figure 10. 20,83% of the labels were incorrectly recognized. It stands out that 8,3% of the error labels result from misinterpreting the white bathroom gown which is worn by the woman who is showering in the video. Another 10% of the error labels represent situations in which many people come together. This assumption is based on the team meetings displayed in the video.
Even though having a lower accuracy rate than Amazon Transcribe, Amazon Rekognition’s tool for detecting labels still convinced me of its usefulness for future research since most of the labels very well described the overall visual content of the video. Knowing the unique labels used in visual storytelling opens up new avenues to apply topic modeling and to explore interrelations between labels themselves as well as in comparison to spoken and/or written narratives. Since the labels come with start and end times, it is also possible to get a deeper understanding of how timing in terms of label detection impacts a video’s attractiveness.
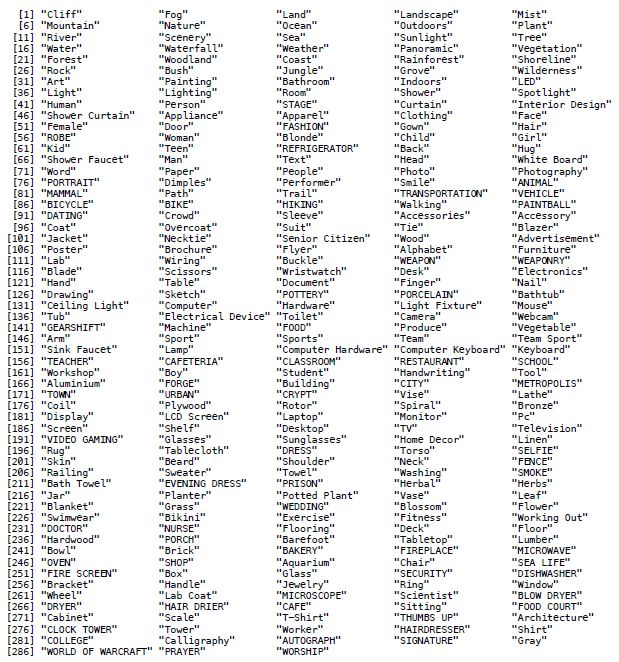
Looking into the Crystal Ball – What the Future May Hold for FRT in General and For Academia in Particular
Accuracy rates are of central importance, especially in fields where based on FRT momentous decisions are made, such as prison sentence or even death penalty. This real threat imposed by FRT, such as Amazon Rekognition, has become omnipresent by the American Civil Liberties Union (ACLU) congress scandal at the latest, where 28 Congress members were falsely matched with persons involved in criminal actions grounded on a confidence level of 80% (Brandom, 2018). ACLU questioned the use of FRT in law enforcement (Snow, 2018). Matt Wood, general manager of AI at AWS, countered with arguing that the confidence level should be set to 90% for public safety issues (Wood, 2018b).
What should be done with a tool that, on the one hand, is able to prevent “human trafficking, inhibiting child exploitation, and reuniting missing children with their families” (Wood, 2018a); on the other hand, it violates privacy rights, discriminates marginalized groups, and has the power to send an innocent person to jail? AWS suggests that to benefit from this powerful tool society needs to prevent misuse of this technology (Wood, 2018b). To ensure this, an established “ethical infrastructure” is required (Floridi, 2013). Otherwise, mistrust might cast a shadow on FRT leverage, resulting in untapped opportunities for improvement, such as using FRT for recognizing objects and alert visually impaired if they become an obstacle for them (Tripathi, Halder, & Vanusha, 2018).
To regulate FRTs’ great power, government, technological companies, and society have to work hand in hand (Blank, 2019). With the help of such a “distributed agency” (Taddeo & Floridi, 2018), regulation is possible. According to Zeng et al. (2019), a responsible use of FRT includes six steps: (1) Improve data privacy, (2) improve accuracy and robustness, (3) reduce bias, (4) inform society, (5) create encrypted test data, (6) constantly check for ethical risks. These steps are congruent with the EU’s AI check list. First movements into this direction have been made in form of the GDPR and the BIPA. There are also first cases were bans and fine issues of FRT were issued (Dave Lee, 2019; Edvardsen, 2019; Fisher, 2019). How the distributed agency ensures the right use of FRT only time will tell. For FRT research not to become “dangerous” and proverbially “deadly”, what Durrenmatt already warned of, also we as researchers have the responsibility to deal with data wisely and should be aware that the use of tools such as Amazon Transcribe and Amazon Rekognition not only processes data but also generates new ones.
For using FRT for academic purposes, I can stress that automating processes through algorithms will facilitate data analysis, especially for larger data sets. On an individual level, errors caused by algorithms can have tremendous consequences, research based on large data sets, however, can prevent a serious distortion of results with the help of confidence values, robustness checks, and random human cross-check, at least in an early stage of machine learning. I believe that when having ensured reliability, services such as Amazon Transcribe and Amazon Rekognition have the power to revolutionize and modernize storytelling research by enabling new approaches which are capable of examining the complex interplay happening in storytelling.
Overall, I think that retelling the methodological story in the field of visual storytelling is of relevance to three groups. Researchers can profit from using innovative methods in order to get a deeper understanding of the power of visual storytelling and learn about how to combine different methodological approaches. Moreover, new insights gained from the use of these innovative methods may help organizations in the future to enhance their performance by improving visual narratives. As a positive side-effect, society may profit from an improved shopping experience.
References
Allison, T. H., Davis, B. C., Short, J. C., & Webb, J. W. (2014). Crowdfunding in a prosocial microlending environment: Examining the role of intrinsic versus extrinsic cues. Entrepreneurship: Theory and Practice, 39(1), 53–73. https://doi.org/10.1111/etap.12108
Allison, T. H., McKenny, A. F., & Short, J. C. (2013). The effect of entrepreneurial rhetoric on microlending investment: An examination of the warm-glow effect. Journal of Business Venturing, 28(6), 690–707. https://doi.org/10.1016/j.jbusvent.2013.01.003
Anda, F., Lillis, D., Le-Khac, N. A., & Scanlon, M. (2018). Evaluating automated facial age estimation techniques for digital forensics. Proceedings – 2018 IEEE Symposium on Security and Privacy Workshops, SPW 2018, 129–139. https://doi.org/10.1109/SPW.2018.00028
Beveridge, J. R., Givens, G. H., Phillips, P. J., Draper, B. A., & Lui, Y. M. (2008). Focus on quality, predicting frvt 2006 performance. IEEE International Conference, 1–8.
Blank, A. L. (2019). Computer Vision Machine Learning and Future- Oriented Ethics.
Brandom, R. (2018). Amazon’s facial recognition matched 28 members of Congress to criminal mugshots. Retrieved from https://www.theverge.com/2018/7/26/17615634/amazon-rekognition-aclu-mug-shot-congress-facial-recognition
Buolamwini, J., & Gebru, T. (2018). Gender Shades: Intersectional Accuracy Disparities in Commercial Gender Classification. Proceedings of Machine Learning Research, 81, 1–15.
Chan, C. S. R., Parhankangas, A., Sahaym, A., & Oo, P. (2019). Bellwether and the herd? Unpacking the u-shaped relationship between prior funding and subsequent contributions in reward-based crowdfunding. Journal of Business Venturing, (April), 1–24. https://doi.org/10.1016/j.jbusvent.2019.04.002
Chouinard, B., Scott, K., & Cusack, R. (2019). Using automatic face analysis to score infant behaviour from video collected online. Infant Behavior and Development, 54(May 2018), 1–12. https://doi.org/10.1016/j.infbeh.2018.11.004
Clarke, J. (2011). Revitalizing Entrepreneurship: How Visual Symbols are Used in Entrepreneurial Performances. Journal of Management Studies, 48(6), 1365–1391. https://doi.org/10.1111/j.1467-6486.2010.01002.x
Conger, K. (2018). Amazon Workers Demand Jeff Bezos Cancel Face Recognition Contracts With Law Enforcement. Retrieved from https://gizmodo.com/1827071904#!
Dave Lee, L. (2019). San Francisco Bans Facial Recognition in US First. Retrieved from www.bbc.com/news/technology-48276660
Davis, B. C., Hmieleski, K. M., Webb, J. W., & Coombs, J. E. (2017). Funders’ positive affective reactions to entrepreneurs’ crowdfunding pitches: The influence of perceived product creativity and entrepreneurial passion. Journal of Business Venturing, 32(1), 90–106. https://doi.org/10.1016/j.jbusvent.2016.10.006
Edvardsen, S. (2019). How to Interpret Sweden’s First GDPR Fine on Facial Recognition in School. Retrieved from iapp.org/news/a/how-to-interpret-swedens-first-gdpr-fine-on- facial-recognition-in-school/
Escalas, J. E. (2004). Imagine Yourself in the Product : Mental Simulation , Narrative Transportation , and Persuasion. Journal of Advertising, 33(2), 37–48.
Fingas, J. (2018). Police Face Recognition Misidentified 2,300 as Potential CriminalsEngadget. Retrieved from https://www.engadget.com/2018/05/06/police-face-recognition- misidentified-2300-as-criminals/
Fisher, C. (2019). Oakland Bans City Use of Facial Recognition Software. Retrieved from https://www.engadget.com/2019/07/17/oakland-california-facial-recognition-ban/?guccounter=1&guce_referrer=aHR0cHM6Ly93d3cuZ29vZ2xlLmNvbS8&guce_referrer_sig=AQAAAJS9qvqVURBnqMcqWMh86nAuwExGTWYKwfXo7L39UmZL-8pThjwuDzgGLv4XnmSn8-3_Mpaugef0ahbJGurHEGqMvKfOj92oRHiRJTg9D9zjYpWmKsfHVL5Mqnq2f3gJ_VIgjmlF9S-xSh0xfeFD_Pp8V9hBluysCMHdPKXZJ8pn
Floridi, L. (2013). Distrubted Moraility in an Information Society. Science and Engineering Ethics, 19(3), 727–743.
Garud, R., Schildt, H. A., & Lant, T. K. (2014). Entrepreneurial Storytelling, Future Expectations, and the Paradox of Legitimacy. Organization Science, 25(5), 1479–1492. https://doi.org/10.1287/orsc.2014.0915
Gehman, J., & Soublière, J. F. (2017). Cultural entrepreneurship: from making culture to cultural making. Innovation: Management, Policy and Practice, 19(1), 61–73. https://doi.org/10.1080/14479338.2016.1268521
Golant, B. D., & Sillince, J. A. A. (2007). The Constitution of Organizational Legitimacy: A Narrative Perspective. Organization Studies, 28(8), 1149–1167. https://doi.org/10.1177/0170840607075671
Hanjalic, A., & Xu, L. Q. (2005). Affective video content representation and modeling. IEEE Transactions on Multimedia, 7(1), 143–154. https://doi.org/10.1109/TMM.2004.840618
Jancsary, D., Meyer, R., Höllerer, M. A., & Boxenbaum, E. (2018). Institutions as multimodal accomplishments: Towards the analysis of visual registers. Research in the Sociology of Organizations, 54A, 87–117.
Jung, S. G., An, J., Kwak, H., Salminen, J., & Jansen, B. J. (2018). Assessing the accuracy of four popular face recognition tools for inferring gender, age, and race. 12th International AAAI Conference on Web and Social Media, ICWSM 2018, (Icwsm), 624–627.
Kaminski, J., Jiang, Y., Piller, F., & Hopp, C. (2017). Do user entrepreneurs speak different? Applying natural language processing to crowdfunding videos. Conference on Human Factors in Computing Systems – Proceedings, 2683–2689. https://doi.org/10.1145/3027063.3053223
Kickstarter. (2020). How to make an awesome video. Retrieved from https://www.kickstarter.com/blog/how-to-make-an-awesome-video
Klare, B., Burge, M., Klontz, J., Bruegge, R., & Jain, A. (2012). Face Recognition Performance: Role of Demographic Information. IEEE Transactions on Information Forensics and Security, 7(6). https://doi.org/10.1109/TIFS.2012.2214212
Knautz, K., & Stock, W. G. (2011). Collective indexing of emotions in videos. Journal of Documentation, 67(6), 975–994. https://doi.org/10.1108/00220411111183555
Kosara, R., & MacKinlay, J. (2013). Storytelling: The next step for visualization. Computer, 46(5), 44–50. https://doi.org/10.1109/MC.2013.36
Kugler, M. B. (2019). From Identification to Identity Theft: Public Perceptions of Biometric Privacy Harms. SSRN Electronic Journal. https://doi.org/10.2139/ssrn.3289850
Laura, A., & Thomi, B. (2019). European Master ’ s Degree in Human Rights and Democratisation The Impact of Biometric Systems at EU Outside Borders on the Human Rights of Irregular Migrating Women.
Levin, S. (2019). “Bias deep inside the code”: the problem with AI “ethics” in Silicon Valley. Retrieved from www.theguardian.com/technology/2019/mar/28/big-tech-ai-ethics- boards-prejudice
Longo, M. (2017). The Politics of Borders: Sovereignty, Security, and the Citizen after 9/11. Cambridge University Press.
Lordkipanidze, O. (2001). The Golden Fleece: Myth, Euhemeristic Explanation and Archaeology. Oxford Journal of Archaeology, 20(1), 1–38. https://doi.org/10.1111/1468-0092.00121
Lounsbury, M., Cornelissen, J., Granqvist, N., & Grodal, S. (2018). Culture, innovation and entrepreneurship. Innovation: Management, Policy and Practice, 21(1), 1–12. https://doi.org/10.1080/14479338.2018.1537716
Lounsbury, M., & Glynn, M. A. (2001). Cultural entrepreneurship: Stories, legitimacy, and the acquisition of resources. Strategic Management Journal, 22(6–7), 545–564. https://doi.org/10.1002/smj.188
Magnet, S. A. (2011). When Biometrics Fail: Gender, Race, and the Technology of Identity. Duke University Press.
Manning, S., & Bejarano, T. A. (2017). Convincing the crowd: Entrepreneurial storytelling in crowdfunding campaigns. Strategic Organization, 15(2), 194–219. https://doi.org/10.1177/1476127016648500
Martens, M. L., Jennings, J. E., & Devereaux Jennings, P. (2007). Do the Stories They Tell Get Them the Money They Need? The Role of Entrepreneurial Narratives in Resource Acquisition. Academy of Management Journal, 50(5), 1107–1132.
Mattern, S. (2018). All Eyes on the Border. Places Journal. https://doi.org/10.22269/180925
Mirza-Babaei, P., Long, S., Foley, E., & McAllister, G. (2011). Understanding the contribution of biometrics to games user research. Proceedings of DiGRA 2011 Conference: Think Design Play, (October).
Mitra, T., & Gilbert, E. (2014). The language that gets people to give. CSCW, 49–61. https://doi.org/10.1145/2531602.2531656
Moss, T. W., Renko, M., Block, E., & Meyskens, M. (2017). Funding the story of hybrid ventures: Crowdfunder lending preferences and linguistic hybridity. Journal of Business Venturing, 33(5), 643–659. https://doi.org/10.1016/j.jbusvent.2017.12.004
Navis, C., & Glynn, M. A. (2010). How New Market Categories Emerge : Radio Satellite. Administrative Science Quarterly, 55, 439–471.
Navis, C., & Glynn, M. A. (2011). Legitimate distinctiveness and the entrepreneurial identity: Influence on investor judgments of new venture plausibility. Academy of Management Review, 36(3), 479–499.
O’Neill, K. (2019). “Facebook’s ‘10 Year Challenge’ Is Just a Harmless Meme- Right?”
Parhankangas, A., & Renko, M. (2017). Linguistic style and crowdfunding success among social and commercial entrepreneurs. Journal of Business Venturing, 32(2), 215–236. https://doi.org/10.1016/j.jbusvent.2016.11.001
Pollack, J. M., Rutherford, M. W., & Nagy, B. G. (2012). Preparedness and cognitive legitimacy as antecedents of new venture funding in televised business pitches. Entrepreneurship: Theory and Practice, 36(5), 915–939. https://doi.org/10.1111/j.1540-6520.2012.00531.x
Resnik, A., & Stern, B. L. (1977). An Analysis of Information Content in Television Advertising. Journal of Marketing, 41(1), 50–53.
Reyes, J., & Bahm, C. R. (2016). Crowdfunding: Applying collective indexing of emotions to campaign videos. Proceedings of the ACM Conference on Computer Supported Cooperative Work, CSCW, 26-Februar, 385–388. https://doi.org/10.1145/2818052.2869075
Roundy, P. (2009). Doing good by telling stories: emotion in social entrepreneurship communication. Journal of Small Business Strategy, 19(2), 41–69.
Sahouria, E., & Avideh, Z. (1999). Content Analysis of Video Using Principal Components. Journal of the American Statistical Association, 9(8), 1290–1298. https://doi.org/10.1080/01621459.1936.10503354
Schwenzow, J., Hartmann, J., Schikowsky, A., & Heitmann, M. (2020). Understanding Videos at Scale : How to Extract Insights for Business Research.
Simonite, T. (2018). When It Comes to Gorillas, Google Photos Remains Blind. Retrieved from https://www.wired.com/story/when-it-comes-to-gorillas-google-photos-remains-blind/
Snow, J. (2018). Amazon’s Face Recognition Falsely Matched 28 Members of Congress With Mugshots. ACLU. Retrieved from https://www.aclu.org/blog/privacy-technology/surveillance-technologies/amazons-face-recognition-falsely-matched-28
Taddeo, M., & Floridi, L. (2018). How AI Can Be a Force for Good. Science, 361(6404), 751–752.
Tripathi, S., Halder, C., & Vanusha, D. (2018). A Survey on Assistive Technology for the Visually Impaired. International Research Journal of Engineering and Technology (IRJET), 1152–1155.
Überbacher, F. (2014). Legitimation of New Ventures: A Review and Research Programme. Journal of Management Studies, 51(4), 667–698. https://doi.org/10.1111/joms.12077
van Werven, R., Bouwmeester, O., & Cornelissen, J. P. (2015). The power of arguments: How entrepreneurs convince stakeholders of the legitimate distinctiveness of their ventures. Journal of Business Venturing, 30(4), 616–631. https://doi.org/10.1016/j.jbusvent.2014.08.001
Wang, M., Deng, W., Hu, J., Tao, X., & Huang, Y. (2018). Racial Faces in-the-Wild: Reducing Racial Bias by Information Maximization Adaptation Network. Retrieved from http://arxiv.org/abs/1812.00194
Wehnert, P., Baccarella, C. V., & Beckmann, M. (2019). In crowdfunding we trust? Investigating crowdfunding success as a signal for enhancing trust in sustainable product features. Technological Forecasting and Social Change, 141, 128–137. https://doi.org/10.1016/j.techfore.2018.06.036
Whitener, M., & Aragon, R. (2019). How should we regulate facial-recognition technology?
Wood, M. (2018a). Amazon general manager of artificial intelligence highlights positive uses of Rekognition & acceptable use policy. Business & Human Rights Resource Center. Retrieved from https://www.business-humanrights.org/en/amazon-general-manager-of-artificial-intelligence-highlights-positive-uses-of-rekognition-acceptable-use-policy
Wood, M. (2018b). Thoughts On Machine Learning Accuracy. Retrieved from https://aws.amazon.com/blogs/aws/thoughts-on-machine-learning-accuracy/
Wry, T., Lounsbury, M., & Glynn, M. A. (2011). Legitimating Nascent Collective Identities: Coordinating Cultural Entrepreneurship. Organization Science, 22(2), 449–463. https://doi.org/10.1287/orsc.1100.0613
Xu, P., Chen, L., & Santhanam, R. (2015). Will video be the next generation of e-commerce product reviews? Presentation format and the role of product type. Decision Support Systems, 73, 85–96. https://doi.org/10.1016/j.dss.2015.03.001
Yang, S., Kher, R., & Newbert, S. L. (2019). What signals matter for social startups? It depends: The influence of gender role congruity on social impact accelerator selection decisions. Journal of Business Venturing, (March). https://doi.org/10.1016/j.jbusvent.2019.03.001
Yavuz, A. (2019). Evaluierung cloudbasierter Machine Learning Services, 49. Retrieved from http://edoc.sub.uni-hamburg.de/haw/volltexte/2019/4531/pdf/Thesis.pdf
Zeng, Y., Lu, E., Sun, Y., & Tian, R. (2019). Responsible Facial Recognition and Beyond. Retrieved from https://arxiv.org/pdf/1909.12935.pdf