Konrad Meisner, University of Siegen, Research assistant at the chair of Entrepreneurship and Family Business
Contact: Konrad.Meisner@uni-siegen.de
Abstract
Constant changes in production, supply chains, and communication influenced our economy and society already manifold. Likewise, Digitalization impacts the present economic environment, similar to automation or electrification in the past. Though the impact and importance of Digitalization in practice and research rises, a common understanding of the terminology cannot be found. While some researchers tried to define buzzwords like Cyber-Physical Systems, Smart Factories, Internet of Things, and many more, there is a lack of consent. Additionally, common standards like ISO or DIN still cannot be found. To ensure a common ground in research, but also for strategic management in companies, this blog entry tries to identify the varying terminology linked to Digitalization, points out differences as well as similarities and leads to a better understanding of the different concepts. The blog entry provides a more in-depth understanding of the technological perspective towards the different concepts and shows linkages between them. The focus is laid on the technologies commonly used in academia, businesses and the news. Thus the concepts of “Internet of Things”, “Cyber-Physical Systems”, “Artificial Intelligence”, “Cloud Computing” and the “Digital Twin” were chosen for further investigation. To achieve a better understanding, various journal articles were reviewed and some definitions were pointed out and discussed. The conclusion finally points out the links between the technologies and the concepts of Digitalization and Industry 4.0. The blog-entry is able to provide thus a common basis for future entrepreneurship research, by building a first glance for researchers and practitioners along.
Digitalization – Why should I bother?
“Fear not the unknown. It is a sea of possibilities.” ― Tom Althouse
Industries and businesses are constantly changing due to technology and progress. Good examples for these changes can be found in the industrial revolutions, like the use of steam machines or electrical appliances. Human strength and long travels were made obsolete by the steam-machine. Likewise, the use of robotics led to an atomized production process since the development of microchips and complex calculations at the end of the century (Gray, 1984). Hermann, Pentek, and Otto (2016) understand the recent stream of Industry 4.0 as one of these disruptive changes in production and logistics which leads to an industrial revolution. While the term was first used by German politics, the term created additional sensation in both academia and businesses with a significant rise in publications. Another term that is used in the case of current technological progress is Digitalization. According to Lasi, Fettke, Kemper, Feld, and Hoffmann (2014) the development of previous digital opportunities, also called digitization, will lead to Industry 4.0. The buzzword “Digitalization” and its interlinked synonyms also receive growing importance in today’s literature (Parida, Sjödin, & Reim, 2019). “Digital Transformation” on the other hand describes how businesses and industries will transform in the near future due to the use of such technologies and has a significant rise since the year 2014 which can be best described as exponentially (Reis, Amorim, Melão, & Matos, 2018). Obviously, Digitalization has already penetrated the view of researchers and practitioners. Entrepreneurial research is not left out on this debate as Nambisan (2017) shows. In his paper, he shows in six different categories, which digital topics and research questions have to be considered and answered in the future. He points out very well, that Digitalization will tackle the existing nature of our understanding of entrepreneurial theories and constructs and it is thus favourable to study this specific phenomenon from various perspectives.
But what exactly is Digitalization? What is not Digitalization? According to my experience, it is not easy to find common ground or a definition of these concepts in Germany. The concept of Digitalization was one of the first that I wanted to research. I aimed to find out what draws businesses to the adaptation of digital technologies. My expectation was to find a new theory on Digitalization or disruptive technologies, but encountered that many business owners lack an understanding of the concept. It became obvious for me that the variety of definitions, the lack of DIN- or ISO-Standards sheds fear among CEOs in my home country. So it became impossible for me to achieve my goals of finding a new theory, so I decided to provide a common ground for researchers and practitioners alike. This blog entry is the second part of a two-part series called “What is what? – Cleaning up the Digitalization mess”, which focuses on the technological side of Digitalization. For the first part which deals with the terminology of the conceptual phrases, see the entry by Kevin Krause. In the following chapters, you will be introduced to different technological concepts. These digital technologies are the “Internet of Things”, “Cyber-Physical Systems”, “Artificial Intelligence”, “Cloud Computing” and the “Digital Twin” concept. These were chosen, as they can be found in various journal articles, the news, and discussions on television, whenever the topic was Digitalization. To build a common ground for future entrepreneurial research, I will discuss some definitions given by academia, by pointing out similarities and differences.
This blog entry can be seen as a first glance towards the concept of Digitalization and tackles three main topics for future entrepreneurship research. First it clarifies and discusses the technologies commonly used in Digitalization. Second, it shows the different technologies in a framework, where all technologies are discussed with its intertwined systems. Lastly this blog-entry delivers a common ground for researchers and practitioners. It can be used by researchers to ensure a common basis prior to quantitative or qualitative surveys in entrepreneurial research, thus ensuring valid results and new knowledge.
Internet of Things – Where does my data come from?
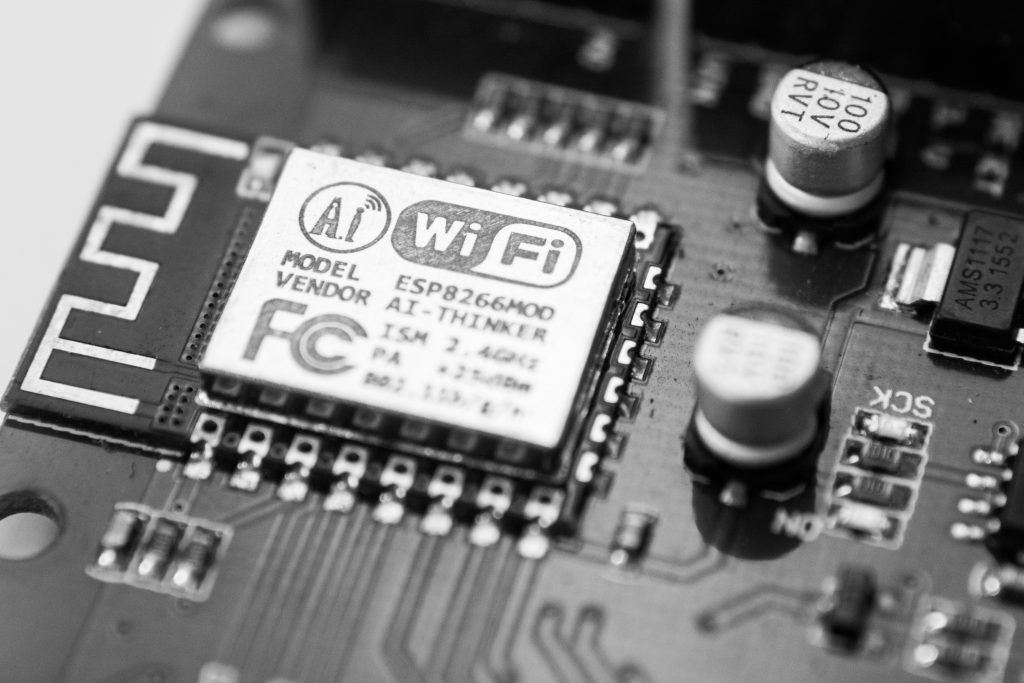
“If you think that the internet has changed your life, think again. The Internet of Things is about to change it all over again!” ― Brendan O’Brien
The “Internet of Things is a concept that shows how the average everyday life and industries are changing due to the application of digital technology within the physical world. The PC is no longer the single port to access the internet and use its possibilities. There are many more access points today than in the past. Society began to use mobile phones and tablets first, but today the main drivers can be found in QR-Codes, GPS, or RFID, which connect the digital world to its twins in the physical world (Khan, Khan, Zaheer, & Khan, 2012). This is generally made possible due to a five-layer system which has been described by Wu, Lu, Ling, Sun, and Du Hui-Ying (2010). The perception layer allows a connection between the physical and digital worlds. Thus measuring physical data and digitizing it. Next, the transportation layer is responsible to send the data to a computer that can process it in the processing layer. This new data then can be used or displayed in the application layer. Last but not least the business layer will have the task to broaden the boundaries of the Internet of Things for future business applications. There are different perspectives on the definition of the Internet of Things such as:
„The Internet of Things (IoT) provides connectivity for anyone at any time and place to anything at any time and place“ (Khan et al., 2012)
The Internet of Things is “Interconnection of sensing and actuating devices providing the ability to share information across platforms through a unified framework, developing a common operating picture for enabling innovative applications. This is achieved by seamless ubiquitous sensing, data analytics and information representation with Cloud Computing as the unifying framework” (Gubbi, Buyya, Marusic, & Palaniswami, 2013)
The Internet of Things is “a conceptual framework that leverages on the availability of heterogeneous devices and interconnection solutions, as well as augmented physical objects providing a shared information base on a global scale, to support the design of applications involving at the same virtual level both people and representations of objects” (Atzori, Iera, & Morabito, 2017)
These three exemplary definitions mainly are connected by the aspect of interconnectivity between the physical and digital worlds. Differences can be found manifold. Atzori et al. (2017) and Khan et al. (2012) both refer to the ubiquitous location of the application while the latter also adds a time perspective to the definition. Otherwise, both definitions seem very act much the same. Gubbi et al. (2013) give a more technical definition of the concept and adds the dimension of being an enabler for innovations. This last aspect is especially interesting in the focus of entrepreneurship research.
Digital Twin – What is my data representing?
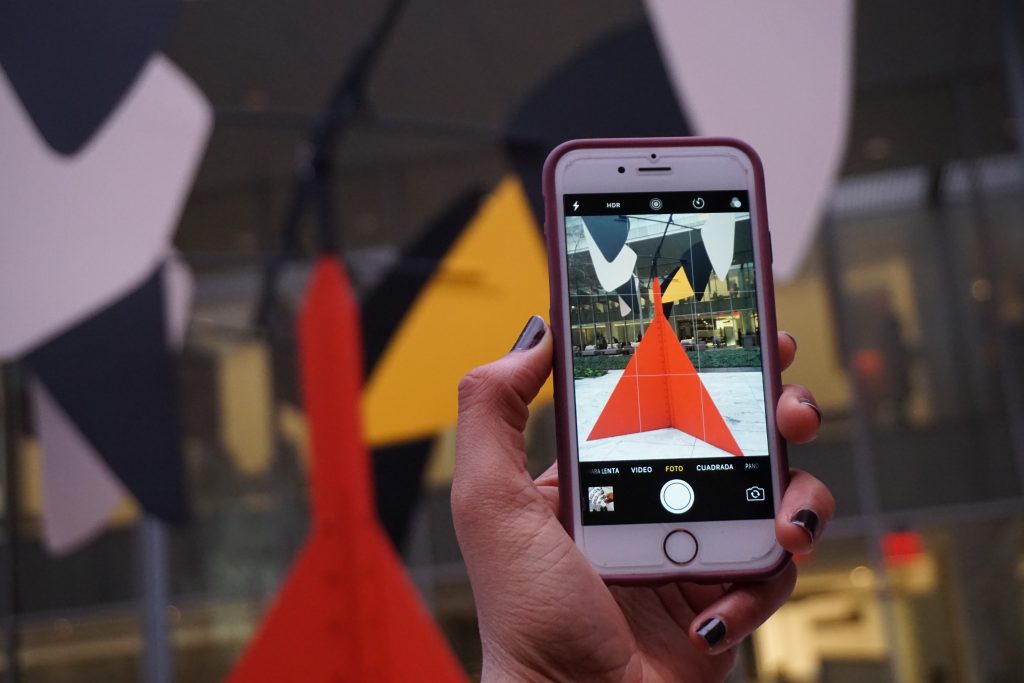
“The truth is we will be forever haunted by traceable communications manifested in the digital world.” ― Germany Kent
The Digital Twin is a concept that can depict a physical entity, like a machine, virtually in such a way, that the gathered data can be perceived as a real-time depiction on a digital basis. The beginning of the use of a Digital Twin can be found especially in production or equipment in a way, that future maintenance could be planned more effectively. Thus it helps to optimize the product lifecycle on a physical basis by using digital data produced by sensors (Tao et al., 2019). Additionally, to the possibility of maintenance enhancement, Digital Twins can be used to enable modularity and autonomy of production systems. This can be achieved by using the technology of the Internet of Things. Sensors and transmission technologies allow better production planning by tracking a machine’s real-time workload and comparing it to the upcoming workload. Thus, understanding the modularity of a machine and comparing if new equipment is needed or could be left out (Rosen, Wichert, Lo, & Bettenhausen, 2015). A good example of the use of Digital Twin technology can be found at NASA. In the paper of Glaessgen and Stargel (2012), it can be found, that the concept will help in future aerospace vehicles. Due to the necessity of more complex systems, materials, and higher risk missions, it will be necessary to have a better understanding of what is happening within a spacecraft. A Digital Twin can be the solution for this problem. How can a Digital Twin be defined? The following definitions give a good understanding of which aspects are crucial for this concept:
“[…] the Digital Twin continuously forecasts the health of the vehicle or system, the remaining useful life and the probability of mission success” (Glaessgen & Stargel, 2012)
“In strict terms, a Digital Twin is a mirror image of a physical process that is articulated alongside the process in question, usually matching exactly the operation of the physical process which takes place in real-time” (Batty, 2018)
“Digital Twin refers to a digital equivalent of physical products, assets, processes, and systems, which is used for describing and modeling the corresponding physical counterpart in a digital manner” (Bao, Guo, Li, & Zhang, 2019)
“DT is characterised by the two-way interactions between the digital and physical worlds, which can possibly lead to many benefits” (Tao et al., 2019)
The last three definitions touch the aspect of mirroring a physical object. Bao et al. (2019) also show, that looking at various aspects of the physical world is important. Thus he proposes, that additional assets, processes, and systems should be regarded more closely. Batty (2018) puts further emphasis on the time aspect and describes, that the mirroring should take place in real-time. Though specifically written for aircrafts, Glaessgen and Stargel (2012)hint towards mission success, while Tao et al. (2019)mention additional benefits, thus the use of Digital Twins is supposed to be beneficial the respective operation, be it a project, production or any other operation.
Cyber Physical Systems – What is businesses data doing?
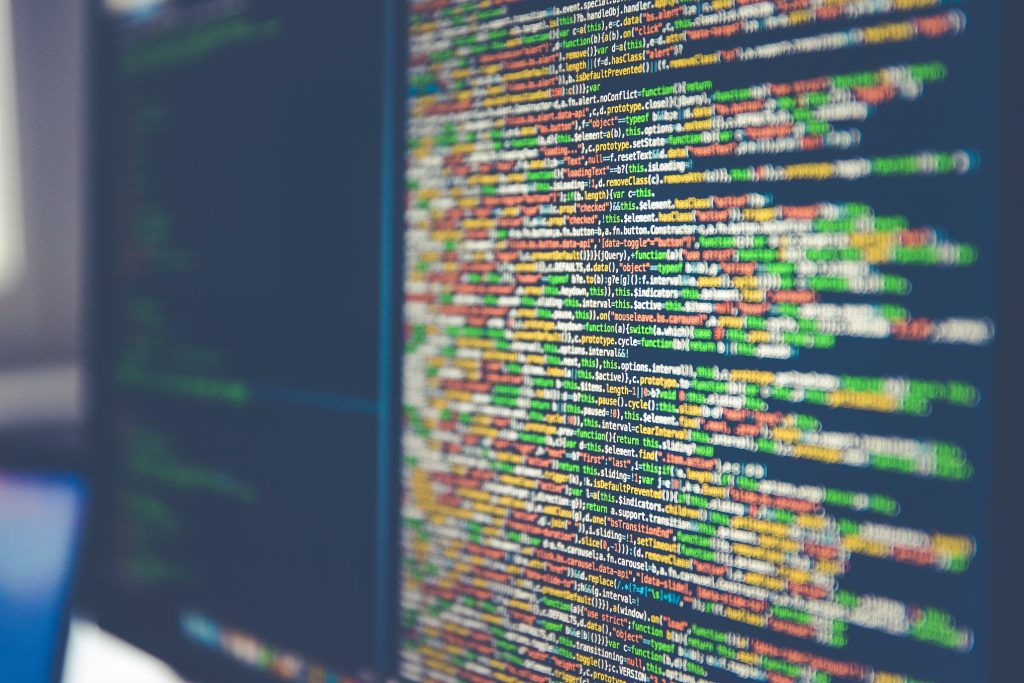
“Combining cyber and physical systems have great potential to transform not only the innovation ecosystem but also our economies and the way we live.” ― Arun Jaitley
The call for a hybrid system that interconnects the layers of the real and digital world had its first call in 1992 (Bakker, 1992). Since then the field of Cyber-Physical Systems has developed significantly. The main problem still is the synchronization between these entities due to physical reasons as the real world is embodied with dynamics, whereas digital information rather can be found in the form of sequences. Still, a five-layer system was developed which describes an architecture for Cyber-Physical Systems. First, the smart connection-level gathers data by using sensors. This data is next processed into digital information on the conversion level which can be then analysed at the cyber level to generate a further call for actions. The cognition level then allows experts to use the data for an optimal decision based on the processed data. Lastly, the data could be used for self-adjustment on the configuration level (Lee, Bagheri, & Kao, 2015). Monostori (2014) sees this system as an enabler for a better connection between people, machines and also products, which shows the difference with the Internet of Things, by directing the concept towards production facilities. Following three exemplary definitions can be found:
“Cyber-Physical Systems (CPS) integrate computing and communication capabilities with monitoring and control of entities in the physical world. These systems are usually composed of a set of networked agents, including sensors, actuators, control processing units, and communication devices” (Cardenas, Amin, & Sastry, 2008)
“Cyber-Physical Systems (CPS) are systems of collaborating computational entities which are in intensive connection with the surrounding physical world and its on-going processes, providing and using, at the same time, data-accessing and data-processing services available on the internet” (Monostori, 2014)
“Cyber-Physical Systems (CPS) is defined as transformative technologies for managing interconnected systems between its physical assets and computational capabilities” (Lee et al., 2015)
These definitions overall are similar in such a way as they refer to the digital representation of physical processes and entities. The essence of these can be found in the definition according to Lee et al. (2015). While Cardenas et al. (2008) refer mostly to the composition of various aspects, Monostori (2014) describes sequences and processes. Though Lee et al. (2015) described in his 5-level system a managerial benefit in the business layer, it cannot be found in his definition. Though that aspect might leverage interest in entrepreneurial activities.
Cloud Computing – What is done to my data?
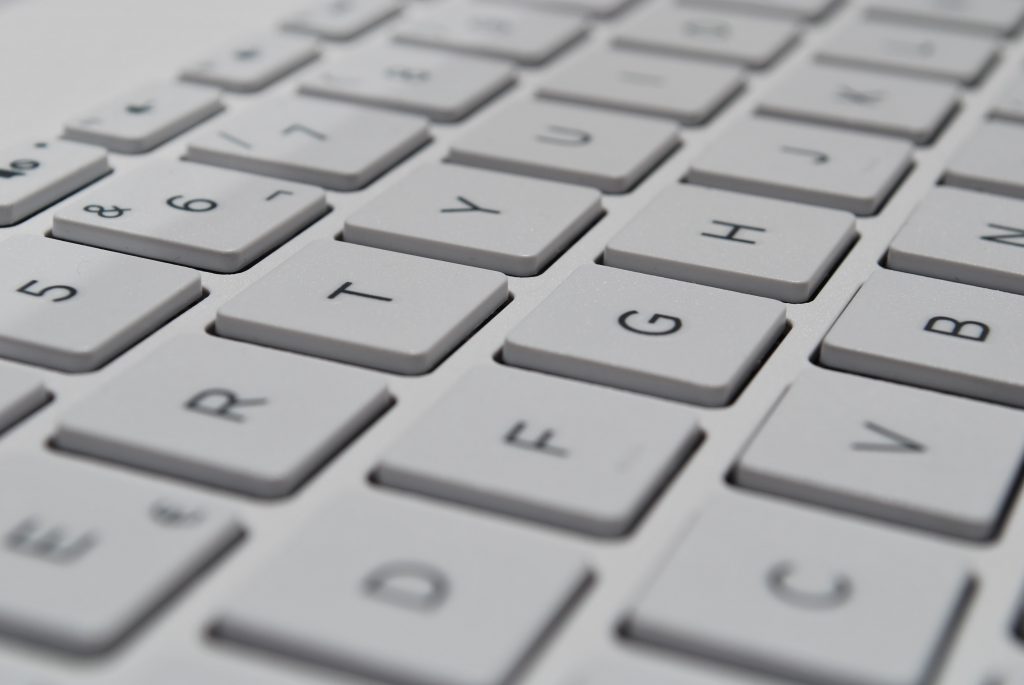
“Never trust a computer you can’t throw out a window” ― Steve Wozniak
Cloud Computing offers new possibilities for data processing. The possibilities and advantages which are generated by using it, are manifold. Advantages can mainly be found in the costs. The user only pays for the use of the booked services. This means, that calculating capabilities can be booked depending on the needs of the user. Additional processing power can be achieved without investing in whole computers or servers. The provider can also link these basics with additional possibilities, which can already be found in common applications. Support, security, and availability are only examples (Kim, 2009). While this may apply to the broad consumer, there is still a lack of trust for businesses. Frauds and corruption of data is too much of a risk. Businesses wish further research before entrusting providers their valuable data either for storage or processing (Sun, Zhang, Xiong, & Zhu, 2014). Several researchers tried to define this concept. Some carefully selected definitions are as following:
Cloud Computing is “being able to access files, data, programs and 3rd party services from a Web browser via the Internet that are hosted by a 3rd party provider and paying only for the computing resources and services used” (Kim, 2009)
“It is an information technology service model where computing services (both hardware and software) are delivered on-demand to customers over a network in a self-service fashion, independent of device and location. The resources required to provide the requisite quality-of-service levels are shared, dynamically scalable, rapidly provisioned, virtualized and released with minimal service provider interaction. Users pay for the service as an operating expense without incurring any significant initial capital expenditure, with the cloud services employing a metering system that divides the computing resource in appropriate blocks” (Marston, Li, Bandyopadhyay, Zhang, & Ghalsasi, 2011)
“Cloud Computing can be considered as a new computing archetype that can provide services on demand at a minimal cost” (Sun et al., 2014)
Marston et al. (2011) provide in their article an interesting but overall important sentence. They state, that there are “as many definitions as there are commentators on the subject”, thus we focus on the selected group of definitions stated above. Kim (2009) restricts himself by stating Cloud Computing services are only accessible by a Web browser, thus excluding all other gateways to the web, otherwise only stating the necessary information on sequences and technology used. On the other hand, the latter definition gets even broader by stating, that it is just a new computing archetype (Sun et al., 2014). Marston et al. (2011) on the other hand gives a very narrow definition and describes technicalities, sequences and even interaction between customer and provider. Overall, every author describes the cost effects of Cloud Computing as an important factor, which can be perceived as a business advantage.
Artificial Intelligence – Friend or Foe?
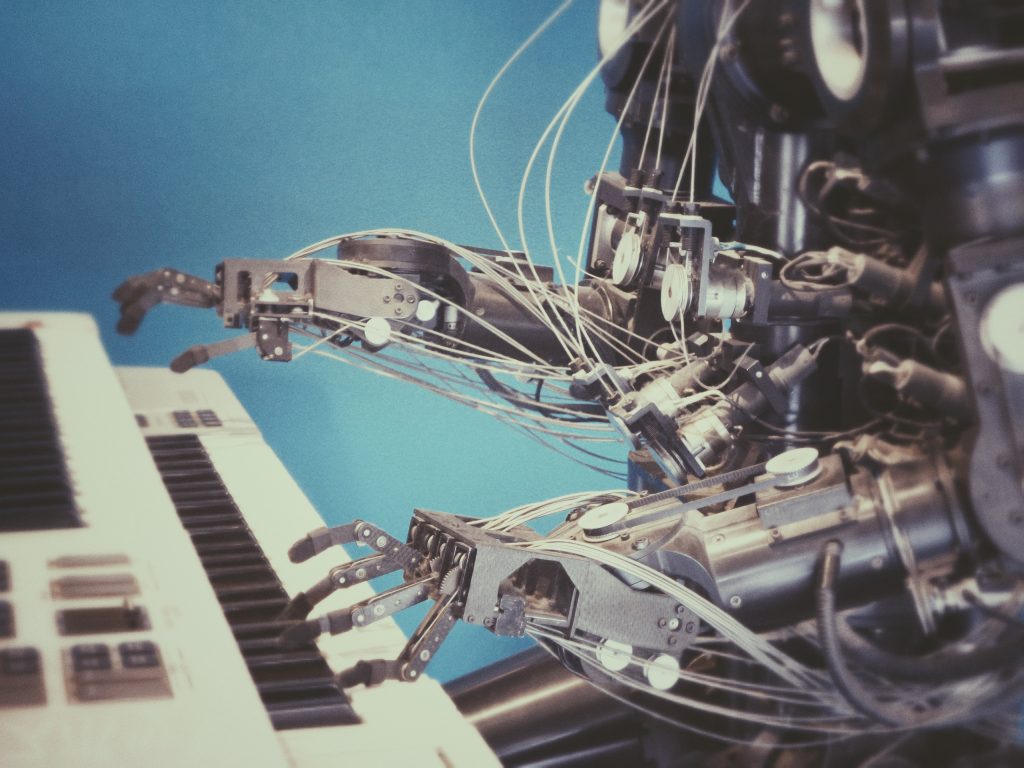
“Before we work on Artificial Intelligence why don’t we do something about natural stupidity?” ― Steve Polyak
Mccarthy, Minsky, Rochester, and Shannon (2006) showed in their proposal for a research project in 1955 the first call for scientific research towards Artificial Intelligence. As an originator of the idea he has posed several questions, like how language could be used to start a thinking process alike human thoughts, how neural networks could be simulated, self-improvement could be obtained or abstract, random, creative processes could be started in machines. This first attempt has been followed by many researchers in different departments, like medicine or warfare, as well as typical business administration fields like transportation or the stock market (Frank, Wang, Cebrian, & Rahwan, 2019). Though being highly discussed, Artificial Intelligence does not receive only positive opinions. Many voices raise doubt in the concept, asking questions like what the spark of human intelligence is and how it can possibly be found in machines (F. Wang, X. Wang, & L. Li, 2016). The question is not new. Turing (1950)already built the question early on. Since 1950 the debate about “true” Artificial Intelligence is going on. Though highly philosophical, Turing (1950)displayed the problem in a test, more likely a game. If a machine was asked different questions which could only be answered by a human, how would it react? Can we anticipate the machine then as a real person? It would get obvious for a person to identify a machine by asking mathematical questions as a machine could calculate the answer way faster than any other person. Thus the question arises on how intelligent a machine is when it is not reacting appropriately in this situation by miscalculating or a similar behaviour (Turing, 1950).
Just recently the “Manager Magazin” published an article about additional controversies towards Artificial Intelligence. They describe that Artificial Intelligence is built upon algorithms that are written and trained by human beings thus it is necessary to look out, not to forget about the individual biases of the programmers. If Artificial Intelligence is trained by a business to reject specific groups of people in employment procedures, is it still beneficial and to whom if so? If the personal bias of the programmer is taken by Artificial Intelligence, is it still intelligent?
We can conclude, that the discussion on this topic raised controversy since the very beginning. How can Artificial Intelligence be defined though? A few examples can be found the following:
“The term Artificial Intelligence denotes behaviour of a machine which, if a human behaves in the same way, is considered intelligent” (A. B. Simmons & S. G. Chappell, 1988)
“AI will be such a program which in an arbitrary world will cope not worse than a human” (Dimiter Dobrev, 2012)
“Artificial Intelligence is the mechanical simulation system of collecting knowledge and information and processing intelligence of universe: (collating and interpreting) and disseminating it to the eligible in the form of actionable intelligence” (Grewal, 2014)
“It is […] referred to as the ability of a machine to learn from experience, adjust to new inputs and perform human-like tasks” (Duan, Edwards, & Dwivedi, 2019)
These different definitions show manifold aspects. The first two presented definitions are philosophical concepts, referring to Artificial Intelligence as the capability to think and act like a human being, but not worse (Simmons and Chappell, 1988; Dimiter Dobrev, 2012). It is interesting, how a bottom-line is defined, but no ceiling. Thus the capabilities of machines are already predefined with the possibility to surpass human intelligence. The fact that technical definitions came way later may derive from the lack of technicality and physical possibilities in the past. Further on a more sequential definition was given by Grewal (2014) and Duan et al. (2019). It seems that Artificial Intelligence has to learn by collecting and processing data and has a pre-step before it could be considered as true intelligent and operate as a human being.
The Turing-Test got passed in 2014 during some experiments by the royal society, specifically conducted experiments by Eugene Goostman. Though latter scientists identified the test to not be sufficient enough. It is merely a demonstration of communication skills, but still lack the proof for other intelligent behaviours (Warwick & Shah, 2016). The show for Artificial Intelligence still will go on.
Conclusion – Now we get somewhere!
“We shall not cease from exploration, and the end of all our exploring will be to arrive where we started and know the place for the first time.” ― T. S. Eliot
As previously described in the introduction of this article, “Internet of Things”, “Cyber-Physical Systems”, “Artificial Intelligence”, “Cloud Computing” and the “Digital Twin” were introduced and discussed. Due to the nature of technologies, they may sometimes seem to be the same, while in fact differing in small details. Using the canvas of Kevin Krause you can find the technologies interlinked in the next picture. Some of the concepts are more related to Industry 4.0, while others fit more to the concept of Digitalization. For further understanding, I highly recommend to read his blog-entry, which is the very foundation of mine.
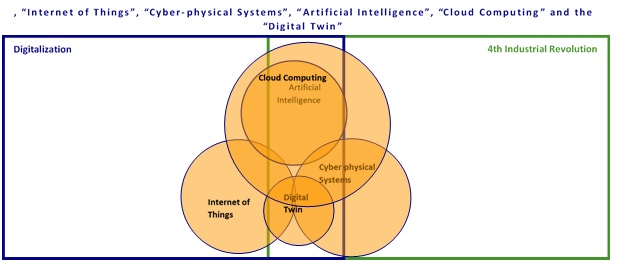
You can find the intertwined systems first confusing, but by looking and analysing closely, the system gets clear. The Internet of Things as previously described gathers data from physical applications and translates these data into a digital language. This digital data can then be used to derive two different concepts. On one hand the data can be translated to a digital twin. A digital twin can be seen as the digital illustration of the physical self. On the other hand, the data can be used in Cyber-Physical Systems to generate strategies or direct commands for other applications. Artificial intelligence in theory can use all of these technologies to derive intelligent new ideas, concepts or strategies by pre-learned algorithms. Thus providing highly beneficial surpluses for a company. All of these concepts can be computed in external networks. Cloud computing thus is providing a platform solely for the use of external computation capabilities, managing the possibility to externalize servers or computers and cutting costs of having to supply own computers or servers.
Though some concepts can be traced back as far as 1950, Digitalization is still in its infancy, but we can easily see a framework, which can be seen in the last paragraph. The technologies that are discussed in this blog-entry are already shaping the landscape of industries, while single business units might not even know about the possible applications. The use of these technologies nevertheless is creating entrepreneurial and innovative potentials. Entrepreneurs and intrapreneurs alike already see possible future applications of such technologies and only wait for the market to be ready for their uses. This blog entry provides the necessary basic knowledge and common ground for researchers and practitioners for future works. Be it for new applications and entrepreneurial ideas in a business environment or for researchers to understand the principles which will change entrepreneurship. In the near future we will see more sophisticated and entrepreneurial use of these technologies. We should be excited about what is awaiting us.
References
Atzori, L., Iera, A., & Morabito, G. (2017). Understanding the Internet of Things: definition, potentials, and societal role of a fast evolving paradigm. Ad Hoc Networks, 56, 122–140. https://doi.org/10.1016/j.adhoc.2016.12.004
B. Simmons, & S. G. Chappell (1988). Artificial intelligence-definition and practice. IEEE Journal of Oceanic Engineering, 13(2), 14–42. https://doi.org/10.1109/48.551
Bakker, J. W. de (Ed.) (1992). Lecture notes in computer science: Vol. 600. Real-time: Theory in practice: Rex Workshop, Mook, The Netherlands, June 3 – 7, 1991 ; proceedings. Berlin: Springer. Retrieved from http://www.loc.gov/catdir/enhancements/fy0815/92014687-d.html
Bao, J., Guo, D., Li, J., & Zhang, J. [Jie] (2019). The modelling and operations for the digital twin in the context of manufacturing. Enterprise Information Systems, 13(4), 534–556. https://doi.org/10.1080/17517575.2018.1526324
Batty, M. (2018). Digital twins. Environment and Planning B: Urban Analytics and City Science, 45(5), 817–820. https://doi.org/10.1177/2399808318796416
Cardenas, A. A., Amin, S., & Sastry, S. Secure Control: Towards Survivable Cyber-Physical Systems. In 2008 The 28th International Conference 6/20/2008 (pp. 495–500). https://doi.org/10.1109/ICDCS.Workshops.2008.40* (Original work published 2008).
Dimiter Dobrev (2012). A Definition of Artificial Intelligence. CoRR, abs/1210.1568.
Duan, Y., Edwards, J. S., & Dwivedi, Y. K. (2019). Artificial intelligence for decision making in the era of Big Data – evolution, challenges and research agenda. International Journal of Information Management, 48, 63–71. https://doi.org/10.1016/j.ijinfomgt.2019.01.021
Wang, X. Wang, & L. Li (2016). Steps toward Parallel Intelligence. IEEE/CAA Journal of Automatica Sinica, 3(4), 345–348. https://doi.org/10.1109/JAS.2016.7510067
Frank, M. R., Wang, D., Cebrian, M., & Rahwan, I. (2019). The evolution of citation graphs in artificial intelligence research. Nature Machine Intelligence, 1(2), 79–85. https://doi.org/10.1038/s42256-019-0024-5
Glaessgen, E., & Stargel, D. (2012). The Digital Twin Paradigm for Future NASA and U.S. Air Force Vehicles. In Structures, Structural Dynamics, and Materials and Co-located Conferences: 53rd AIAA/ASME/ASCE/AHS/ASC Structures, Structural Dynamics and Materials Conference (22267B). [Place of publication not identified]: [publisher not identified]. https://doi.org/10.2514/6.2012-1818
Gray, H. J. (1984). The New Technologies: An Industrial Revolution. Journal of Business Strategy, 5(2), 83–85. https://doi.org/10.1108/eb039061
Grewal (2014). A Critical Conceptual Analysis of Definitions of Artificial Intelligence as Applicable to Computer Engineering. IOSR Journal of Computer Engineering, 16, 9–13. https://doi.org/10.9790/0661-16210913
Gubbi, J., Buyya, R., Marusic, S., & Palaniswami, M. (2013). Internet of Things (IoT): A vision, architectural elements, and future directions. Future Generation Computer Systems, 29(7), 1645–1660. https://doi.org/10.1016/j.future.2013.01.010
Hermann, M., Pentek, T., & Otto, B. (2016). Design Principles for Industrie 4.0 Scenarios. In T. X. Bui & R. H. Sprague (Eds.), Proceedings of the 49th Annual Hawaii International Conference on System Sciences: 5-8 January 2016, Kauai, Hawaii (pp. 3928–3937). Piscataway, NJ: IEEE. https://doi.org/10.1109/HICSS.2016.488
Khan, R., Khan, S. U., Zaheer, R., & Khan, S. [Shahid] (2012). Future Internet: The Internet of Things Architecture, Possible Applications and Key Challenges. In 10th International Conference on Frontiers of Information Technology (FIT), 2012: 17 – 19 Dec. 2012, Islamabad, Pakistan ; proceedings (pp. 257–260). Piscataway, NJ: IEEE. https://doi.org/10.1109/FIT.2012.53
Kim, W. (2009). Cloud Computing: Today and Tomorrow. The Journal of Object Technology, 8(1), 65. https://doi.org/10.5381/jot.2009.8.1.c4
Lasi, H., Fettke, P., Kemper, H.‑G., Feld, T., & Hoffmann, M. (2014). Industrie 4.0. WIRTSCHAFTSINFORMATIK, 56(4), 261–264. https://doi.org/10.1007/s11576-014-0424-4
Lee, J., Bagheri, B., & Kao, H.‑A. (2015). A Cyber-Physical Systems architecture for Industry 4.0-based manufacturing systems. Manufacturing Letters, 3, 18–23. https://doi.org/10.1016/j.mfglet.2014.12.001
Marston, S., Li, Z., Bandyopadhyay, S., Zhang, J. [Juheng], & Ghalsasi, A. (2011). Cloud computing — The business perspective. Decision Support Systems, 51(1), 176–189. https://doi.org/10.1016/j.dss.2010.12.006
Mccarthy, J., Minsky, M., Rochester, N., & Shannon, C. E. (2006). A Proposal for the Dartmouth Summer Research Project on Arti cial Intelligence. AI Magazine, 27.
Monostori, L. (2014). Cyber-physical Production Systems: Roots, Expectations and R&D Challenges. Procedia CIRP, 17, 9–13. https://doi.org/10.1016/j.procir.2014.03.115
Nambisan, S. (2017). Digital Entrepreneurship: Toward a Digital Technology Perspective of Entrepreneurship. Entrepreneurship Theory and Practice, 41(6), 1029–1055. https://doi.org/10.1111/etap.12254
Parida, V., Sjödin, D., & Reim, W. (2019). Reviewing Literature on Digitalization, Business Model Innovation, and Sustainable Industry: Past Achievements and Future Promises. Sustainability, 11(2), 391. https://doi.org/10.3390/su11020391
Reis, J., Amorim, M., Melão, N., & Matos, P. (2018). Digital Transformation: A Literature Review and Guidelines for Future Research. In Á. Rocha, H. Adeli, L. P. Reis, & S. Costanzo (Eds.), Advances in Intelligent Systems and Computing: Volume 745. Trends and advances in information systems and technologies: Volume 1 (Vol. 745, pp. 411–421). Cham: Springer International Publishing. https://doi.org/10.1007/978-3-319-77703-0_41
Rosen, R., Wichert, G. von, Lo, G., & Bettenhausen, K. D. (2015). About The Importance of Autonomy and Digital Twins for the Future of Manufacturing. IFAC-PapersOnLine, 48(3), 567–572. https://doi.org/10.1016/j.ifacol.2015.06.141
Sun, Y., Zhang, J. [Junsheng], Xiong, Y., & Zhu, G. (2014). Data Security and Privacy in Cloud Computing. International Journal of Distributed Sensor Networks, 10(7), 190903. https://doi.org/10.1155/2014/190903
Tao, F., Sui, F., Liu, A., Qi, Q., Zhang, M., Song, B., . . . Nee, A. Y. C. (2019). Digital twin-driven product design framework. International Journal of Production Research, 57(12), 3935–3953. https://doi.org/10.1080/00207543.2018.1443229
Turing, A. (1950). Computing Machinery and Intelligence. Mind, LIX, 433–460.
Warwick, K., & Shah, H. (2016). Passing the Turing Test Does Not Mean the End of Humanity. Cognitive Computation, 8, 409–419. https://doi.org/10.1007/s12559-015-9372-6
Wu, M., Lu, T.‑J., Ling, F.‑Y., Sun, J., & Du Hui-Ying (2010). Research on the architecture of Internet of Things. In D. Wen (Ed.), 3rd International Conference on Advanced Computer Theory and Engineering (ICACTE), 2010: 20 – 22 Aug. 2010, Chengdu, China ; proceedings (V5-484-V5-487). Piscataway, NJ: IEEE. https://doi.org/10.1109/ICACTE.2010.5579493